Are you trying to pick between the top data cloud platforms? The Databricks vs Snowflake debate is key for companies wanting better data solutions.
Businesses today need strong cloud data platforms. Databricks and Snowflake are leaders, turning data into useful insights.
I’ll show you the strengths of these platforms. We’ll look at their differences and uses in different fields. Knowing Databricks and Snowflake’s features will help you choose the best for your data needs.
Table of Contents
Key Takeaways
- Databricks and Snowflake represent cutting-edge cloud data platforms
- Each platform offers unique architectural approaches to data processing
- Performance, scalability, and integration capabilities differ significantly
- Choosing the right platform depends on specific organizational needs
- Both platforms support advanced analytics and machine learning workflows
Understanding Cloud Data Platform Fundamentals
Cloud data platforms have changed how businesses handle big data. They give companies tools to turn data into useful insights.
At their heart, cloud data platforms are a smart way to handle big data. They let businesses quickly store, process, and analyze data.
Defining Cloud Data Platforms
A cloud data platform has key features:
- It can grow with more data
- It works well with many data sources
- It can process data fast
- It keeps data safe and follows rules
Evolution of Data Processing Solutions
Data processing has come a long way. It started with old databases and now uses cloud systems. Companies always look for better ways to handle data.
- 1990s: Local server-based databases
- 2000s: First cloud storage
- 2010s: Big data analytics platforms
- 2020s: AI-powered data systems
Key Components of Modern Data Platforms
Today’s cloud data platforms have many parts. They include tools for big data, machine learning, and analytics. These tools help turn data into important business insights.
“Data is the new oil, and cloud platforms are the refineries of the digital age.” – Tech Industry Insight
Also Read: What is Snowflake Database
An Introduction to Databricks and Snowflake
Databricks and Snowflake are changing the game in cloud data lakes. They help organizations manage and analyze huge amounts of data. This is a big deal for data engineering tools.
Databricks made a big splash by bringing together data engineering and machine learning. It was started by the creators of Apache Spark. This powerful platform lets data scientists and engineers work together smoothly.
- Provides a collaborative data science workspace
- Supports multiple programming languages
- Integrates advanced machine learning capabilities
Snowflake changed cloud data warehousing with its unique design. It keeps storage and compute separate. This lets businesses grow their data easily. Their cloud-native approach makes data management simpler and faster.
“We built Snowflake to solve the limitations of traditional data warehousing approaches.” – Frank Slootman, CEO of Snowflake
Both platforms tackle big challenges in data engineering:
- Handling massive data volumes
- Enabling real-time analytics
- Providing scalable infrastructure
- Supporting advanced data processing techniques
As more companies make decisions based on data, Databricks and Snowflake are key. They turn raw data into useful insights.
Core Architectural Differences
Cloud data platforms change how we handle big data. Databricks and Snowflake use new ways to manage and analyze data.
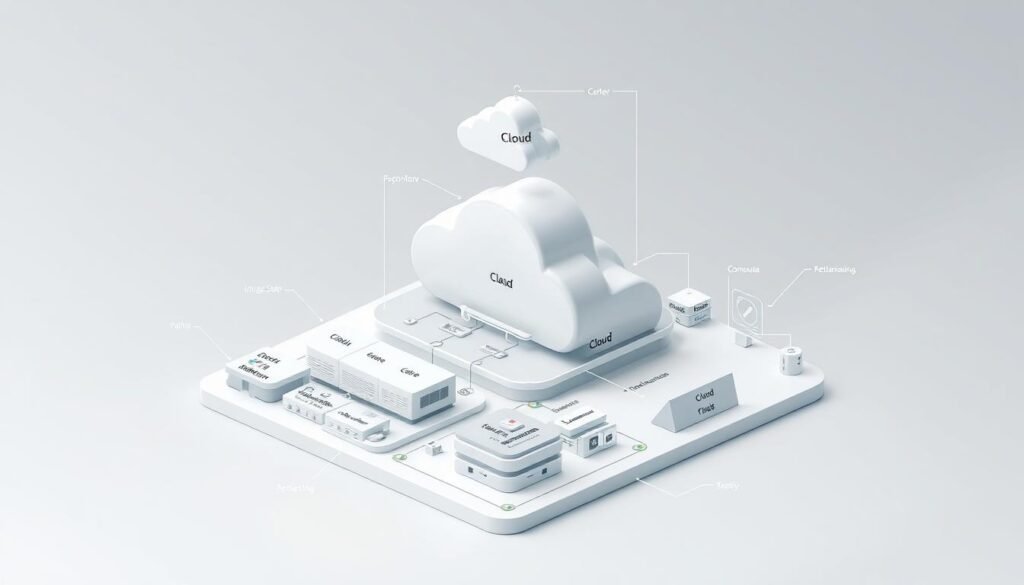
The design of data platforms affects their speed and flexibility. Each platform has its own way to tackle big data challenges.
Databricks Lakehouse Architecture
Databricks has a new lakehouse architecture. It combines data lakes and warehouses. This makes it easier to manage all kinds of data.
- Unified data management across structured and unstructured data
- Direct access to raw data files
- Enhanced support for machine learning workflows
- Seamless integration of analytics and data engineering
“The lakehouse paradigm represents a transformative strategy for handling diverse data ecosystems.” – Data Architecture Expert
Snowflake’s Multi-Cluster Architecture
Snowflake has a multi-cluster shared-data architecture. It makes data processing fast and scalable. It separates storage and computing.
- Independent scaling of storage and compute
- Dynamic resource allocation
- Automatic optimization of query performance
- Reduced infrastructure complexity
Storage and Compute Separation
Both platforms separate storage from computing. This lets companies use data storage better. It saves money and makes systems more flexible.
Databricks and Snowflake show the future of data processing. They give companies tools to turn data into useful information.
Also Read: Advantages of Snowflake
Performance and Scalability Capabilities
Databricks and Snowflake are top choices for big data. They show how well they handle lots of data. Let’s look at what makes them different.
Snowflake is known for its flexibility. It lets you scale storage and compute separately. This makes it easy to manage data without hassle.
- Instant resource allocation
- Seamless horizontal scaling
- Automatic performance optimization
Databricks is fast because of its special design. It mixes data warehousing and lakes. This gives users control over their data.
Feature | Snowflake | Databricks |
---|---|---|
Scaling Capability | Instant Elastic Scaling | Granular Resource Control |
Compute Separation | Full Separation | Configurable Separation |
Workload Optimization | Automatic | Manual Configuration |
Both platforms are great for big data. Snowflake is easy to use. Databricks is customizable for specific needs.
Data Processing and Analytics Features
Looking at Databricks and Snowflake, we see big differences in how they handle data. They each have special ways to deal with complex data tasks. This shows their unique strengths in managing data.
Today’s businesses need strong data solutions. Databricks and Snowflake offer new ways to tackle tough data problems.
Real-time Processing Capabilities
Databricks uses Apache Spark’s strong engine for fast data processing. It has great features like:
- Streaming data ingestion
- Complex event processing
- Unified analytics across batch and streaming workflows
Batch Processing Options
Snowflake is great for SQL-based batch processing. It has a special setup for:
- Automatic query optimization
- Scalable computational resources
- Efficient large-scale data transformations
Query Performance Optimization
Both platforms have top-notch query optimization. Databricks uses distributed computing for quick complex queries. Snowflake uses smart caching to boost speed.
The choice between Databricks and Snowflake depends on specific organizational data processing requirements and analytics strategies.
Also Read: What Companies Use Snowflake
Databricks vs Snowflake: Direct Comparison
The debate between Databricks and Snowflake shows big differences. These differences affect how companies handle their data. Each platform has its own strengths, making the choice important and thoughtful.
I looked at what makes these data solutions different. I focused on their main features and how they work.
Comparison Criteria | Databricks | Snowflake |
---|---|---|
Primary Strength | Advanced data science and machine learning | Enterprise data warehousing |
Architectural Model | Lakehouse architecture | Multi-cluster shared data architecture |
Processing Capabilities | Real-time and batch processing | Optimized for structured data queries |
Snowpark vs Databricks Support | Extensive MLflow integration | Native SQL-based data processing |
The comparison shows how Databricks and Snowflake handle data differently. Databricks is great for complex data science tasks. Snowflake is best for traditional analytics.
- Databricks excels in machine learning and AI-driven projects
- Snowflake specializes in scalable data warehousing
- Both platforms support multi-cloud deployments
Choosing between Databricks and Snowflake depends on your needs. It’s about what your company needs, how you process data, and your data strategy for the future.
Integration and Ecosystem Support
Data cloud platforms change how we handle big data. They offer great integration. Databricks and Snowflake make it easy to connect with many tools and places.
Third-party Tool Integration
Databricks and Snowflake are great at working with many data tools. Databricks uses Apache Spark. Snowflake has a marketplace for easy tool sharing.
- Business Intelligence (BI) tool connections
- Data visualization platforms
- Machine learning frameworks
- ETL and data transformation tools
Cloud Provider Compatibility
Being able to use different clouds is important. Databricks and Snowflake work with big cloud providers. This lets companies use their favorite cloud.
Cloud Provider | Databricks Support | Snowflake Support |
---|---|---|
Amazon Web Services | Full Support | Full Support |
Microsoft Azure | Full Support | Full Support |
Google Cloud Platform | Full Support | Full Support |
API and Connector Options
APIs help developers make custom tools. Databricks and Snowflake have good REST APIs and special connectors. This helps with big data.
“Integration is the key to unlocking the full data cloud platforms.” – Data Analytics Expert
Choosing between Databricks and Snowflake depends on what your company needs. Look at your ecosystem and technology.
Security Features and Compliance Standards
When looking at cloud data storage, security is key. Databricks and Snowflake focus on keeping data safe. They use strong data rules to protect important info.
Both platforms have strong security steps. They keep data safe with:
- Advanced end-to-end encryption protocols
- Multi-factor authentication systems
- Granular access control mechanisms
- Real-time threat detection capabilities
They also follow important rules to keep data safe. Industry-standard certifications show they care about protecting data:
Compliance Standard | Databricks | Snowflake |
---|---|---|
GDPR | ✓ | ✓ |
HIPAA | ✓ | ✓ |
SOC 2 | ✓ | ✓ |
Knowing about these security steps helps companies protect their data. Databricks and Snowflake use top data rules. This keeps cloud data safe and sound.
Pricing Models and Cost Considerations
Finding the right data warehousing solution is key. Databricks and Snowflake have different pricing plans. These plans can affect your budget for handling big data.
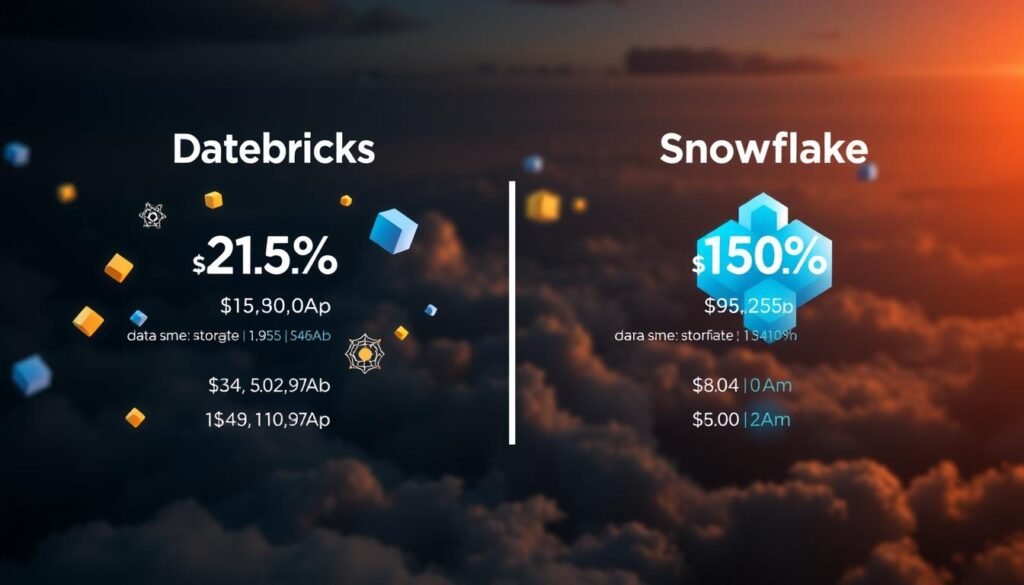
Cloud data platforms change how we handle and analyze data. It’s important to know the pricing details to make smart choices.
Understanding Usage-Based Pricing
Both platforms have flexible pricing:
- Snowflake charges based on what you use
- Databricks has both subscription and usage-based plans
- Prices change based on how much you need
Cost Optimization Strategies
To save money, try these:
- Use automated scaling
- Watch and learn from your usage
- Use reserved instances for better deals
Platform | Pricing Model | Cost Optimization |
---|---|---|
Snowflake | Consumption-based | High granularity, pay-per-second |
Databricks | Hybrid pricing | Flexible scaling options |
Hidden Costs and Considerations
Don’t just look at the price. Other costs like data transfer, storage, and complex queries can add up.
“Understanding the total cost of ownership is more important than comparing headline pricing rates.” – Data Architecture Expert
By studying these pricing models, you can pick the best data warehousing solution. This will help you handle big data without breaking the bank.
Machine Learning and AI Capabilities
Data engineering tools like Databricks and Snowflake have changed how we do machine learning and AI. They give data science teams strong tools to work with.
Databricks is known for its big machine learning system. It works well with many ML frameworks. It also has MLflow for tracking and managing experiments.
- Native ML workflow support
- Integrated experiment tracking
- Scalable computational resources
- Support for popular ML frameworks
Snowflake uses partnerships and new ways to work with machine learning. It’s not as built-in as Databricks but helps a lot with getting data ready for ML.
Feature | Databricks | Snowflake |
---|---|---|
ML Framework Support | Native Integration | Partner Ecosystem |
Experiment Tracking | MLflow | Limited Native Tools |
Computational Scalability | High | Moderate |
When picking between Databricks and Snowflake, think about what your project needs. Databricks is great for direct ML work. Snowflake is better for getting data ready.
Both Databricks and Snowflake are really working hard to make machine learning and AI better in cloud data engineering.
Data Governance and Management Tools
Data governance is a big challenge for today’s companies using cloud data integration. Databricks and Snowflake have different ways to manage and protect data. Each has its own strengths.
Databricks and Snowflake have different data management plans. Databricks uses Delta Lake, an open-source layer. It offers:
- ACID transactions for data reliability
- Schema enforcement capabilities
- Time travel for data versioning
- Robust data quality controls
Snowflake has a strong access control system. Its platform has:
- Granular role-based access controls
- Advanced data sharing capabilities
- Secure data collaboration features
- Integrated compliance monitoring
Both platforms care about data integrity. But they use different ways to do it. Databricks uses Delta Lake for reliability. Snowflake focuses on secure data sharing and access.
Effective data governance is no longer optional—it’s a strategic imperative for data-driven organizations.
Companies need to think hard about these data integration frameworks. They should look at their compliance needs, data processing, and future goals. This helps choose between Databricks and Snowflake.
Use Cases and Industry Applications
Cloud data lakes change how companies handle data. Snowpark vs Databricks is key for finding the best data solutions. This is true for many industries.
Today’s businesses use these tools to find deep insights. They make big decisions based on this. Each industry needs its own data handling, so picking the right cloud is very important.
Enterprise Analytics Solutions
Finance, healthcare, and retail get a lot from cloud data lakes. They use them for deep analytics. The benefits are:
- They can process data fast
- They grow with big tasks
- They work well with machine learning
Data Science Workflows
Data scientists use Databricks and Snowflake for complex tasks. Collaborative environments help teams:
- Build smart machine learning models
- Do big data changes
- Use predictive analytics
Business Intelligence Applications
Business intelligence teams use cloud data lakes for insights. They get tools for:
- Making interactive dashboards
- Creating detailed reports
- Showing data in cool ways
Knowing Snowpark and Databricks’ strengths helps companies improve their data plans. This leads to innovation in many fields.
Conclusion
In the world of cloud data platforms, Databricks and Snowflake stand out. They offer strong solutions for data analytics. Each has its own strengths for different needs.
Choosing between them depends on what your organization needs. Databricks is great for complex data and machine learning. Snowflake shines in data warehousing and fast queries. Think about your current setup, growth needs, and future data plans.
Remember, there’s no one-size-fits-all solution. Teams should look at things like how easy it is to integrate, costs, and what you need to do. The market keeps changing, with Databricks and Snowflake leading the way.
Choosing the right platform is key for success. It can make your business more efficient and competitive. Be open to change, keep up with new tech, and see platform choice as a big decision for your business.
FAQ
What is the main difference between Databricks and Snowflake?
Databricks combines data lakes and warehouses in one place. It’s great for analytics and machine learning. Snowflake is a cloud-native data warehouse. It’s best for SQL-based analytics and data warehousing.
Which platform is better for machine learning and data science?
Databricks is better for machine learning and data science. It has tools for ML and supports many programming languages. Snowflake is more for traditional data warehousing and has less ML.
How do the pricing models of Databricks and Snowflake differ?
Both use usage-based pricing but differently. Snowflake charges for storage and compute separately. Databricks bundles these and has more complex pricing. Each has ways to save money.
Can I use both Databricks and Snowflake together?
Yes, many use both together. Databricks handles complex data and ML. Snowflake is the main data warehouse. They work well together.
Which platform is more suitable for big data processing?
Databricks is great for big data. It uses Apache Spark and handles large datasets well. Snowflake is good for structured data but not as strong for very large datasets.
What are the key security features of these platforms?
Both have strong security. Databricks has access control and encryption. Snowflake has more, like multi-factor authentication and end-to-end encryption.
How do Databricks and Snowflake handle data integration?
Databricks has lots of integrations and supports many data formats. Snowflake has a data marketplace and many connectors. Both work with big cloud providers but differently.
Which platform is more cost-effective?
It depends on what you need. Snowflake is often cheaper for simple data warehousing. Databricks is better for complex tasks that need advanced computing.
What cloud providers do Databricks and Snowflake support?
Both support AWS, Azure, and Google Cloud. Databricks has tight integration with each. Snowflake also supports these, making it easy to deploy and move data.
How do these platforms handle real-time data processing?
Databricks is better for real-time processing with Spark streaming and Delta Live Tables. Snowflake is improving but is more batch-oriented. Databricks is more flexible for streaming data.